Generalization in Deep Learning
Author(s)
Kawaguchi, Kenji; Kaelbling, Leslie Pack; Bengio, Yoshua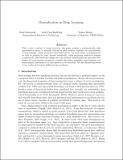
Downloadgedl.pdf (355.7Kb)
Other Contributors
Learning and Intelligent Systems
Advisor
Leslie Kaelbling
Terms of use
Metadata
Show full item recordAbstract
With a direct analysis of neural networks, this paper presents a mathematically tight generalization theory to partially address an open problem regarding the generalization of deep learning. Unlike previous bound-based theory, our main theory is quantitatively as tight as possible for every dataset individually, while producing qualitative insights competitively. Our results give insight into why and how deep learning can generalize well, despite its large capacity, complexity, possible algorithmic instability, nonrobustness, and sharp minima, answering to an open question in the literature. We also discuss limitations of our results and propose additional open problems.
Date issued
2018-05-01Series/Report no.
MIT-CSAIL-TR-2018-014
Keywords
neural network, learning theory
Collections
The following license files are associated with this item: