Towards Understanding Generalization via Analytical Learning Theory
Author(s)
Kawaguchi, Kenji; Benigo, Yoshua; Verma, Vikas; Kaelbling, Leslie Pack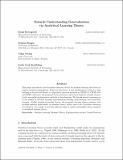
DownloadMIT-CSAIL-TR-2018-019.pdf (383.3Kb)
Metadata
Show full item recordAbstract
This paper introduces a novel measure-theoretic theory for machine learning
that does not require statistical assumptions. Based on this theory, a new
regularization method in deep learning is derived and shown to outperform
previous methods in CIFAR-10, CIFAR-100, and SVHN. Moreover, the proposed
theory provides a theoretical basis for a family of practically successful
regularization methods in deep learning. We discuss several consequences of
our results on one-shot learning, representation learning, deep learning,
and curriculum learning. Unlike statistical learning theory, the proposed
learning theory analyzes each problem instance individually via measure
theory, rather than a set of problem instances via statistics. As a result,
it provides different types of results and insights when compared to
statistical learning theory.
Date issued
2018-10-01Series/Report no.
;MIT-CSAIL-TR-2018-019
Keywords
Machine Learning, Measure Theory, Regularization method, Neural Network